After obtaining his PhD from Dublin City University (DCU) in 2011, Dr Rejwanul Haque entered into the language technology industry and worked on industrial machine translation (MT) solutions for seven years.
He re-joined DCU’s MT team in 2018 and worked as an industry-oriented postdoc at SFI’s Adapt research centre. Since January 2019, he has been working as a research fellow with a Marie SkÅ‚odowska-Curie Fellowship.
His research is supported by the Euraxess Hosting Agreement Scheme, which enables approved research enterprises to recruit experts from outside the European Economic Area for their R&D departments in Ireland.
‘In cases where language is a barrier to access of pertinent information, MT may help people assimilate information published in different languages’
– DR REJWANUL HAQUE
What inspired you to become a researcher?
Prior to my PhD, I obtained my degree from Jadavpur University, India, where I worked as a research engineer with the Ministry of Communication and Information Technology. This was part of a sponsored consortia-based project, ‘cross-lingual information access’ (CLIA), for two years.
From that time, I confronted many profound challenges in relation to the project and worked on different natural language processing (NLP) problems such as parts-of-speech tagging, named entity recognition and MT. My interest in this area of research grew out over time during my tenure in the CLIA project.
Can you tell us about the research you’re currently working on?
I primarily work on MT, which is arguably regarded as the most difficult problems scientists could ever contemplate doing on a computer.
These problems include terminology translation, knowledge distillation, interactive MT, low-resource MT, data selection and domain adaptation. Although my primary research area is MT, my interests also include other NLP problems such as question-answering, social media analytics and information extraction.
In your opinion, why is your research important?
Every day more people are becoming infected and dying across the world due to Covid-19 pandemic. In cases where language is a barrier to access of pertinent information, MT may help people assimilate information published in different languages.
As part of the DCU MT team, we have recently built eight multilingual MT engines that are specifically trained to translate Covid-19 material between German, French, Italian, Spanish into English, as well as the reverse direction.
We have enabled online public access to the systems where users can select their source and target languages via a drop-down menu, and paste their desired text into the source panel. The language-appropriate MT server carries out the translation, and the translation is instantaneously retrieved to appear in the source panel.
We have already published this research in ArXiv with the hope of contributing to the fight against Covid-19 and to have a direct impact on society.
What commercial applications do you foresee for your research?
The current state-of-the-art neural approaches to MT typically require millions of parallel sentences and powerful large-scale clusters or GPUs for training, which has been viewed as a ‘non-green’ technology. The cost for GPUs is too high, making many SMEs unable to deploy this cutting-edge innovation in their translation pipeline.
Also, use of large data increases training and experiment time, making it more difficult for MT users such as translation service companies and MT researchers.
Our technology would help SMEs or individual users to select a small but representative training data for building [neural machine translation] systems on resource-limited devices to provide high-quality services. In other words, our research helps reduce the MT training costs.
What are some of the biggest challenges you face as a researcher in your field?
In recent years, we have witnessed the change of MT technology from statistical methods to deep learning methods, with higher demands on computing and data resources, such as powerful hardware and massive amounts of parallel data.
For example, Google recently built a massive multilingual neural MT system with 25bn-plus sentence pairs and 50bn-plus model parameters.
Many SMEs are unable to afford such computing resources, and this prohibits them to deploy this technology in their production. This is also a problem in academia as most of the research institutes cannot afford such computing resources.
Are there any common misconceptions about this area of research?
Nowadays, there are many concerns over the fact that MT poses a threat to the services that professional translators currently offer. However, it would never be the case that the MT systems would generate error-free translations one day. It will always make some mistakes, and never replace the professional translators who would always be the essential part of the industrial translation workflows.
What are some of the areas of research you’d like to see tackled in the years ahead?
Term translation is a well-known problem in MT research. A suitable solution to integrate terminology into MT would certainly impact the translation industry and be a breakthrough in MT research.
Neural MT training can benefit from large-scale data, although this has many downsides. It relies on large-scale powerful hardware such as GPUs. The cost for such hardware is quite high, which makes many SMEs unable to afford these resources.
Selecting a smaller representative subset from large-scale training data would speed up training and lower computation cost, especially benefiting SMEs who have limited computational resources and use neural MT in their production.
Are you a researcher with an interesting project to share? Let us know by emailing editorial@siliconrepublic.com with the subject line ‘Science Uncovered’.
The post How machine translation can help bring Covid-19 info to the masses appeared first on Silicon Republic.
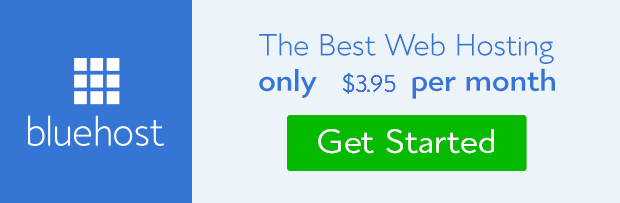
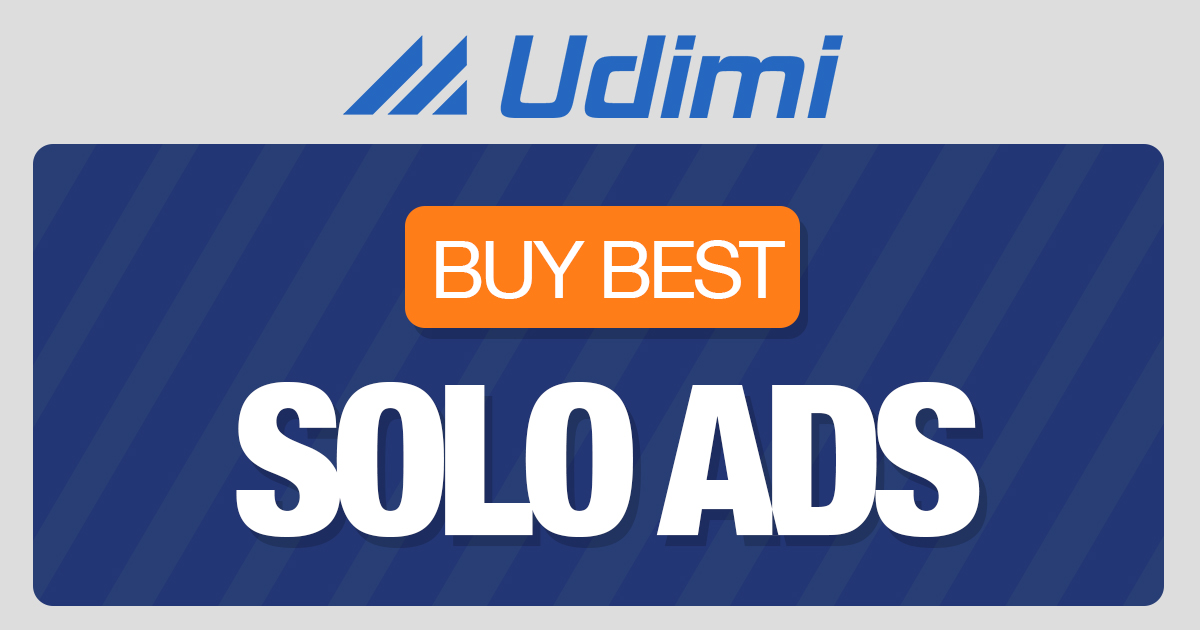
via IFTTT
Comments
Post a Comment